Fundamental Factors That Improve Your Investments
Our take on Lausberg, Slager, and Stork's framework for measuing and steering fundamental factors in a portfolio.
For most institutions, asset allocation decisions are the gentle tweaks that steer an investment plan away from the choppy waters of risk. Risks are mapped to fundamental drivers of return which tie to the macroeconomy: growth, inflation, illiquidity, and volatility (Ilmanen, 2011). These changes require judgment, and that judgement has increasingly been aided by data and tooling.
Beyond the judgment of the captain, the most pressing questions are often what data should be used and how should allocators leverage the most out of this data from the available tooling. A recent paper on this subject in the Journal of Investing caught our attention, in part because it is both a collaboration of academics and an institutional investor. Pim Lausberg, sr. portfolio manager at APG, alongside colleagues Alfred Slager, professor at TIAS, Tilburg, and Philip Stork, professor at VU University, Amsterdam, seek to provide a practical solution to this question in their paper, “Fundamental Factors That Improve Your Investments: A Practical Guide.” They (collectively “LSS”) provide a practical approach to improving asset allocation decisions by simplifying the data conundrum around asset class and factor exposures.
A Mapping Exercise
Exposures are the foundation of any analysis, and LSS begin with a foundational assumption that asset allocators think about exposure in asset allocation buckets. We see this broadly across our clients. They simplify exposures into eight asset classes, but also decompose them through the lens of nine core factors. These factors are not as complex as factors like Fama-French factors, and intentionally so. This allows the allocator with no more data than an asset allocation model to map correlations between their asset allocation framework and a fundamental understanding of factor exposure, which can ultimately tie back to their understanding of the drivers of future return. At the very least, this simplifies the equation of understanding a base-level comprehension of risk, even if it could be improved (more on that later). They think this is particularly important because, “a combination of factors should not cause undesirable secondary effects.”
By simply looking at a historical lookback of returns, allocators can map their exposures to these factors in order to better understand their cross-asset class correlations and a rough sense of the ensuing “factor” exposures. These factors ultimately translate into those drivers of return. The following correlation matrix (Figure 1) from the paper provides a snapshot at these correlations in domestic asset classes across a twenty year period:
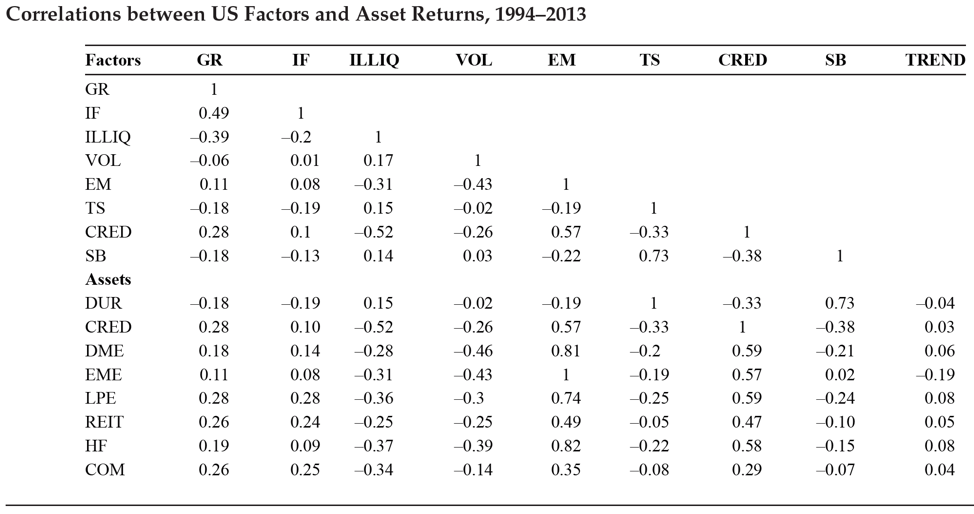
Steering the Ship
Let’s assume that we, an allocator, map our asset allocation framework to these asset classes and then to the fundamental factors listed above. From there, we determine the correlation of our capital at risk to various factors. What’s next? Well for one, the allocator now has an understanding of unintended factor exposures, which cut across asset classes, with the goal of ultimately mapping these to the drivers of returns.
In doing this, Ilmanen proved that the first four factors in the list above (GR = growth, IF = inflation, ILLIQ = illiquidity and VOL = volatility) are those which—empirically and theoretically—explain most of the cross-sectional variation of returns. Hence, they are the primary to consider.
This exercise is particularly important because most decisions to tilt a portfolio are driven by a macroeconomic projection of how those fundamental drivers of return will fare in the future. As LSS write, this is functionally useful for understanding the unintended exposure:
“Rather than changing the strategy–setting process from one focused on asset classes to one focused on factors, candidate asset allocations can be checked for “unwanted” factor exposures. Should an investor desire more or less exposure to a certain factor, the asset allocation can be adjusted appropriately.”
Figure 2 provides LSS’ example of a pension plan rebalancing its existing exposure (“SAA”) from an overweight on fixed income to a tactical exposure (“TAA”), which overweights developed market equities, hedge funds, and duration, away from bonds. This decision may be informed by the macro-perspective the allocator has with regard to fixed income, or say, inflation.
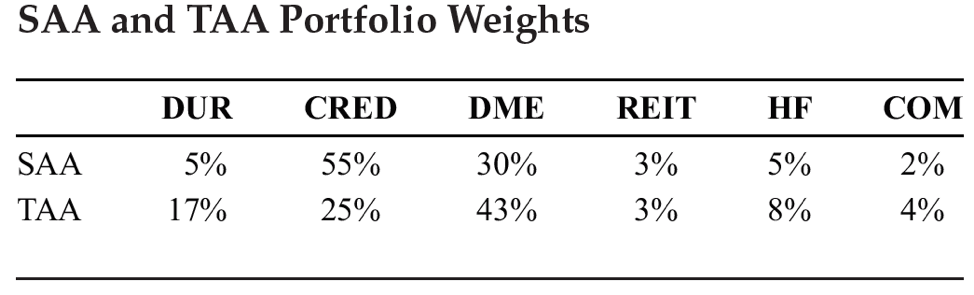
Through LSS’ framework, the allocator is provided an additional perspective toward the change in their portfolio’s exposure through the lens of factor exposures. These factor exposures can then be mapped to the fundamental factors which drive return, namely the aforementioned growth, illiquidity, inflation, and volatility. With some basic assumptions around factor covariances, we can see how the aggregate ‘factor sensitivity’ of the portfolio changes with this rebalancing from SAA to TAA. For posterity, the authors also include a further decomposition of term spread and credit spread given the change to the fixed income allocation in Figure 3:
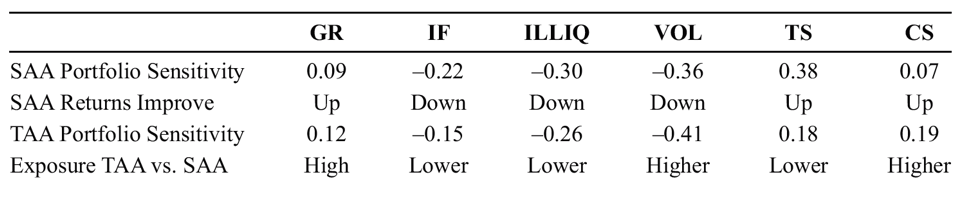
This decomposition provides a clearer understanding of macroeconomic views with the consequences of rebalancing asset classes.
Much of this is intuitive and not predicated on lofty assumptions. Growth sensitivity increases, while term spread decreases. Credit spread increases as a function of duration increasing, even though the headline ‘bond’ allocation decreases. These nuanced perspectives offer the risk committee a better sense of how these sweeping changes impact the portfolio should it find itself in a different inflation, duration, or growth environment.
An Enriching Twist
At Novus, we are firm believers in bottoms-up exposure aggregation rather than asset allocation decompositions of risk. By that we mean we like to understand the “devil in the details“ behind asset allocations. For example, a hedge fund portfolio could be viewed as a homogenous asset class whose behavior is predicated on historical correlations of an alternative index’s returns, or it can be understood fundamentally through the asset class and directional exposure at the individual manager and aggregated up to the asset allocation level.
In turns out that—based on a decade-long work with allocator clients using exposure data submitted by their underlying hedge funds for asset allocation purposes—the exposure data, when used accurately through tools like Novus, can enhance the predictive power for future returns.
By doing this, the allocator can also better understand true risk exposure, as a function of excess net exposure, geographic, or single-name tilt. Of course, this requires more data accumulation, cleansing, and aggregation, which is our core value proposition for allocators.
But that doesn’t mean that the approach of LSS conflicts with our perspective. In fact, we believe in combining their practical model of measuring and mapping cross-asset correlation with our ability to provide a more nuanced understanding of the true exposures underlying each asset class. By computing true exposure bottoms-up, the asset allocation framework is improved and thus the cross-asset correlations become sharper. Unintended risks can be better averted.
Another argument in favor of combining the two approaches—as opposed to seeing them as antithetic—is that the correlation matrices underlying the fundamental factor approach discussed here are unstable in the short term. Saying that a correlation between factors is negative over a long, 20-year period says nothing over the short-term. To prove the point, recall what happened to the historically positive long-term correlation between equity returns and treasury yields during 2010-2015: it flipped, and remained negative for a long time, under the distortions of a world fueled by quantitative easing. Since you can’t rely on correlations in the short term, you might as well look at raw exposures to asset class as an adjunction to your investment decision process, something our clients do.
LSS cite a paper by Ang, Goetzmann, and Schaefer (2009), which “reported that the Norwegian Government Pension Fund was relatively strongly exposed to changes in two factors, namely volatility and illiquidity, which spiked up during the financial crisis of 2007–2009. Because the Norwegian Fund appeared not to have been fully aware of these exposures, the ensuing large losses came as a negative surprise.”
This is something we have seen broadly across institutional investors leading into 2008. Many dealt with margin calls and have taken years to retrench their illiquid exposures back into their asset allocation frameworks. We believe that by implementing sensible frameworks of decomposing risk, as described by LSS, while enriching it with bottoms-up data aggregation, investors can improve the efficacy of their tactical asset allocation decisions. In a sense, they can have their cake and eat it too.