Diving for Skill: How to Fight Mean Reversion
Position-level analysis helps investors uncover manager skill and avoid the traps of selection bias, behavioral bias, and style drift.
What tools are available to institutions for evaluating active managers? Broadly, there are three. The first is qualitative inference, which considers people, process, and pedigree. The bedrock of investing is trust, so this is a crucial aspect of constructing strong bonds between allocator and manager. Think of it as the interview section of the college application. You’re getting to know someone with whom you want to forge a long-lasting relationship.
The next two tools are quantitative, split between analyzing results (returns) and input variables (security-level holdings). This is like evaluating GPA versus the nuances of a candidate’s transcript. One is a single, distilled numerical value, while the other unpacks that number’s base-level ingredients, providing a more specific array of strengths and weaknesses (good at chemistry, terrible at history!). Both tools are important but draw insight differently. Like GPA for college admission, returns are the currency here, because in the end they are what matters. Unlike a college application, they are often the only quantitative data provided—and thus the lowest common denominator of skill set evaluation. Imagine admitting a student on two top-level numbers (SAT, GPA) and an interview where the candidate talks about how great they are!
But what can we tell about the skill of an investment manager through the positions supporting their results? It all depends on tooling. Like a transcript, one can achieve more granular decomposition of achievement through holdings with profit/loss with that tooling. At Epsilon Asset Management, we focus singularly on revealing the skill sets of active managers through holdings-based analysis. We also test them for predictive value (more on that later). These are methods of analysis that we were fortunate to incubate at Novus, working with some of the brightest capital allocators and institutional investment managers. We believe skill set analysis provides an opportunity to better distinguish alpha from beta, tilting the odds of capital allocation decisions through robust statistical inference (more on that later as well). Given the pervasive nature of mean reversion in capital allocation decisions, allocators need every advantage making selections, and position-level analysis can help.
Mean Reversion
Why is mean reversion such a problem for capital allocation? Three reasons: selection bias, behavioral bias, and style drift. I’ll briefly unpack each before explaining how position-level skill set analysis can help ameliorate these headwinds.
Selection Bias
Let’s say you’re fortunate to work at a premier university endowment. In the institutional landscape, you’re the belle of the ball. The world’s best investment managers covet your capital, because it is infinitely sticky. Perhaps more so, it signifies to the broader community a sense of acceptance through securing an allocation. You have the pick of the litter. If returns were the sole quantitative element of your analysis, you wouldn’t even bother reviewing opportunities that underperform because—all else equal—you have access to top performers.
Here’s the problem: by cutting the distribution of eligible managers in half (assuming a bell-curve distribution by performance), your sample of outperformers includes both skilled as well as lucky managers. Just from a simple statistical standpoint, some percentage of investment managers with great returns was simply lucky, and you can bet your life savings that they will craft a compelling narrative around that outperformance. It’s now your job to differentiate true skill, even though both parties are doing their best to qualitatively infer their skill.
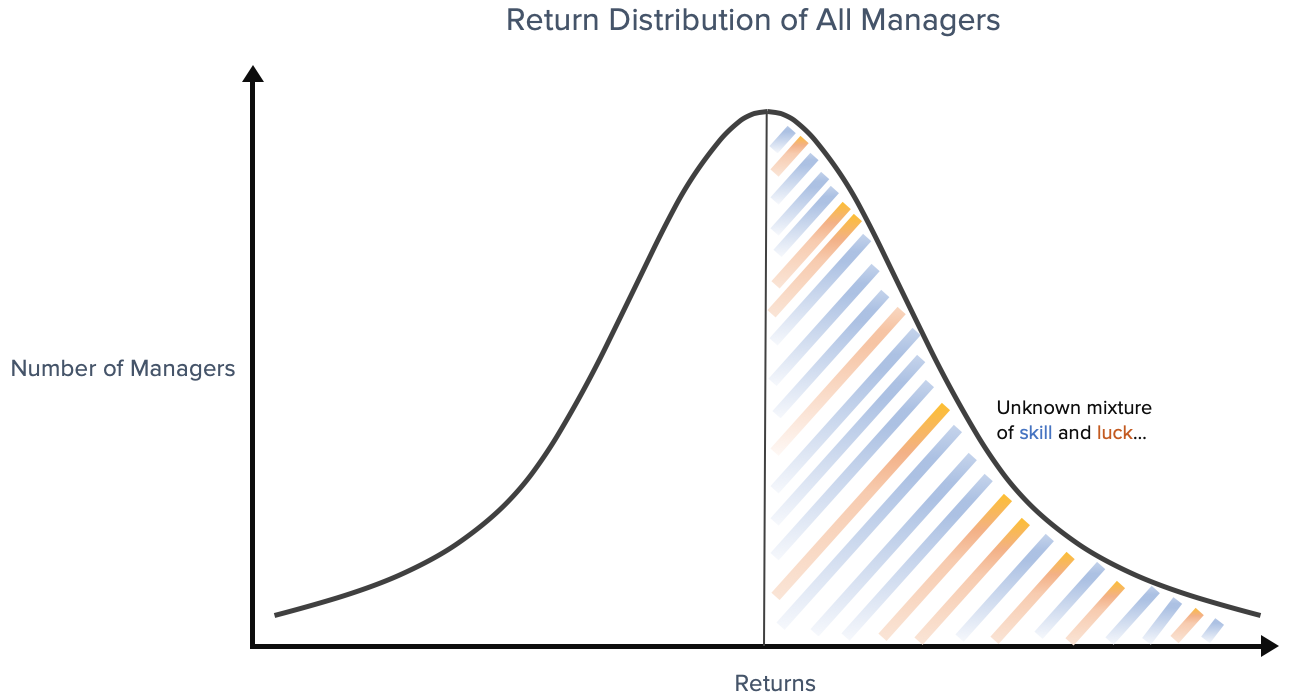
This is not a straightforward task. Many world-renowned capital allocators I’ve worked with lean on the qualitative to do so. They’ve framed their job as “investigative journalism” because they must constantly source, verify, and validate information. The unknown mixture of skill and luck is a reality of a game played in a complex adaptive system with wide distributional outcomes. Every box in your diligence kit can be checked, but that manager may mean revert the day after you write them a check. This leads to frustrating feedback, because results aren’t a clear indication of decision making. Process must become the focus.
Even with the best process, some percentage of lucky managers will make their way into institutional portfolios. The lucky alphas are prone to mean revert, and because they are drawing from the right side of the distribution, those reversions will by and large be negative. This is selection bias at its core.
Behavioral Bias
A lot of ink has been spilled in the realm of behavioral finance, and with good reason. Humans are not well equipped through their evolutionary process to make decisions that bypass fight/flight reactions. It’s part of what makes capital markets so exciting (or gut-wrenching) with their sentiment-driven moves. Sources of excitement, like gambling, can be bad for us, and the evidence is clear with capital allocation.
Study after study shows that allocating to investments that have cyclical components (as most do) leads investors to buy tops and sell bottoms. Think of any investing cycle, whether it be a commodity like gold or a sector such as software. The information that signifies the relative attractiveness in that investment permeates through markets, creating feedback loops that can lead to speculative bubbles. Making allocations to fund managers isn’t much different. Certain studies show that alpha produced by fund managers is often a function of these feedback loops, wherein success begets flows. Flows are reinvested in existing investments, pushing their values up.
Style Drift
Style drift is a function of a few disparately connected phenomena that can roughly be bucketed under the “diseconomies of scale” of asset management. If your investment strategy is niche or unscalable, your success will draw flows. That flow can dilute one’s edge if it is capacity constrained. It’s very hard for an investment manager to say no to capital inflows, given the nonlinear profitability of the business as a function of scale. And so, too often, managers accept fund flows and dilute what we at Epsilon call their circle of alpha generative competence. They may be competent at ancillary pursuits (e.g., the high-frequency quant shifting into the neighboring practice of stat arb), but it may not be the focal point of their edge.
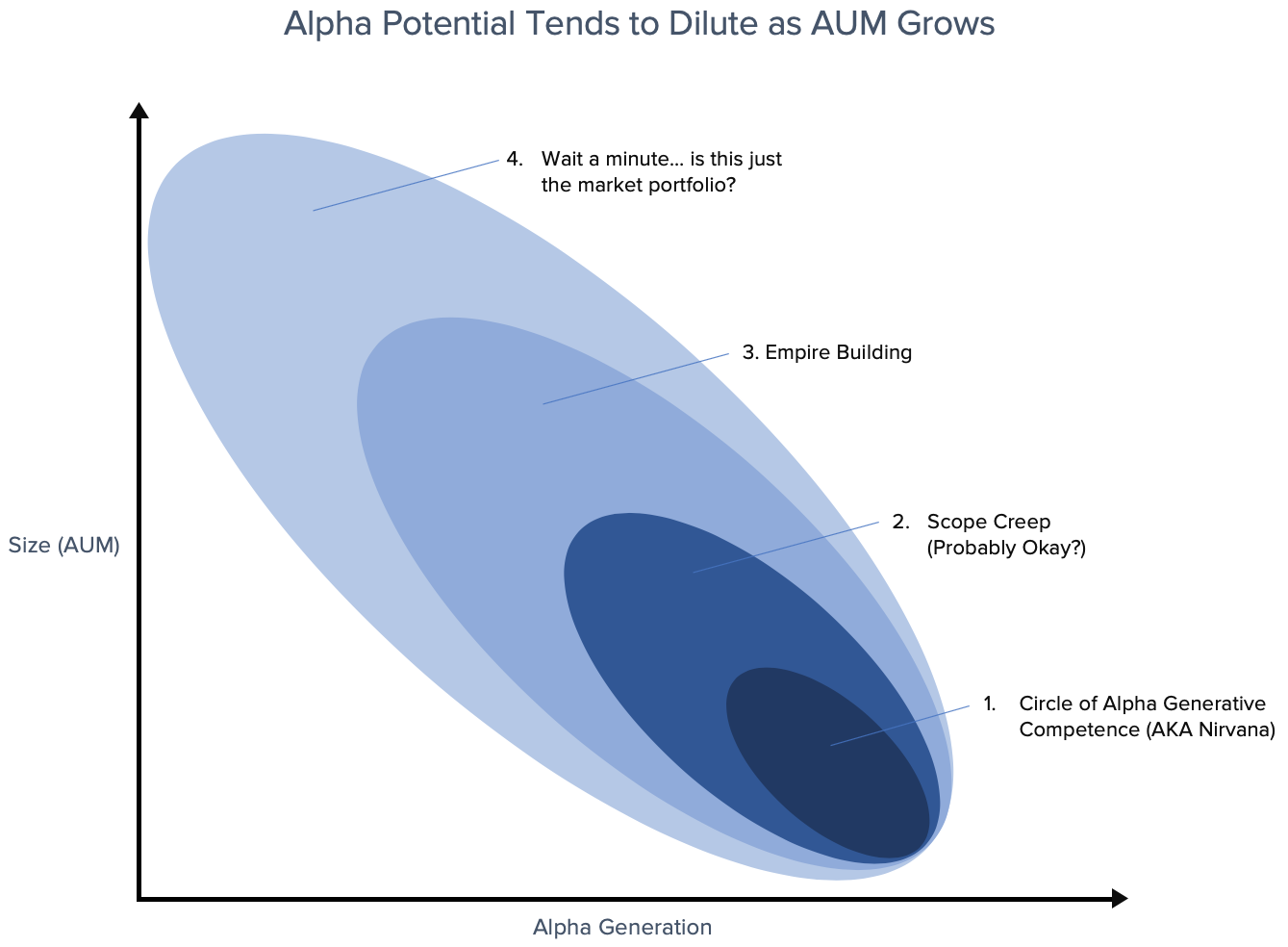
Scaling problems are the least of style drift concerns. Too often, the fantastical renumeration of our industry can lead to lifestyle changes for fund principals who reevaluate the quality of life associated with endless security analysis. On the other end of the spectrum, empire building can become a problem when scale causes organizations to rapidly increase headcount, technology, offices, and counterparties, only to find that business management may be less their forte than investment management.
Holdings-Based Analysis: A Suit of Armor
What we painted above is bleak, especially when an investor focuses on returns as a means for making capital allocation decisions. Thankfully, many allocators are fantastic at qualitative inference, which helps combat these headwinds. But too often they’re light on holdings-based analysis. This was historically a function of available technology and actually achieving transparency. Thankfully, the trend on both has been positive in recent years. SaaS solutions like Novus, or in-house stacks built on the primitives of cloud compute and open-source calculation libraries have proliferated at a rapid pace. The ability to draw insight from “big data” is a much simpler exercise than in years past.

We posit that holdings-based analysis allows one to better infer the ingredients that elicit skill versus simply looking at returns. It should act as a check for biased narrative and red flags. There are several vectors of revealed skill that we as a firm focus on. Solutions like Novus do a great job providing ready-to-go analytics across many of these. For the purpose of this piece, we’ll highlight one: analyzing skill through security selection and how that adds to the probability of capital allocation success.
Security Selection
It’s one of the most tangible skills that we pay investment managers for. It’s often the skill that investment managers talk about the most (especially on the short side), because it encapsulates their area of primary focus: security analysis. We have to be careful about inferring security selection skill through dialogue. Narratives are drawn after the fact for why a manager fortuitously was long security X while short security Y. From an empirical standpoint, we want to determine if all of their choices demonstrate repeatable and statistically significant skill. We cannot determine this either through returns or qualitative inference. The former will conflate other ingredients of return (e.g., portfolio construction, trading, luck). It would be like determining if a student was better at art history or chemistry from an SAT score. At best, there may be some tenuous connection. The narrative will be carefully selected by the manager (highlight the winners, forget the losers).
Holdings-based analysis allows us to decompose security selection through a variety of frameworks at the granular level of trade-level data. For those that like factor-based models, regressions can be run at the individual constituent level and aggregated to the total portfolio. This is advantageous to portfolio-level return regression, because it can allow for flexible calculation parameters. For example, say a domestic manager has significant exposure to Chinese technology. If you regress her returns against a domestic factor model (simply because they are a so-called domestic manager), your model’s definition of alpha may spuriously attribute betas to alpha. The only way to tackle that holistically is through constituent-level analysis.
Further, you may not believe in factor models and would rather think about asset allocation as a function of opportunity cost. Tools such as Novus Framework (a flexible opportunity cost model calculated at the constituent level) or Brinson Frameworks allow for comparable means of stripping out alpha from beta. Each of these tools provides a slightly different lens of insight in the quantification of skill.
The Predictive Power of Holdings-Based Alpha vs Returns
At Epsilon, we test the predictive value of skill sets through statistical analysis. You can think of that as an alternative risk premia we hope to capture, because we intuit that it is a more robust predictor than simple returns.
This is easier said than proven, so let’s show you an example. We’re going to run a little experiment across 2,000 fund managers and their publicly disclosed equity positions through ownership data capture. If you’ve read this blog, it’s a pretty common topic of analysis. Given its public nature, we’ll leverage it for the sake of this analysis. Its primary strength is the statistical significance of the dataset, without problems such as survivorship or selection bias. Veritably any hedge fund that’s filed 13Fs in the last twenty years is present in this example.
So here’s the experiment: we will run a trailing four quarter vs. forward four quarter correlation for every manager in our investment universe across two metrics: top level return and security selection alpha. That allows us at a very basic level to understand if one has a higher predictive power than the other. We’ll test this across 2,000 separate institutions and twenty years of ownership data. This represents approximately eight million individual fund-level positions over time and a peak of over three trillion dollars of filled assets.
What did we find? Skill exhibited much lower variance and far less mean reversion than simple top-level return did. If you’re curious regarding the methodology, feel free to reach out, and I can take you to the nethervoid of statistical analysis. Sparing the rest of you, we found statistically significant superiority in combating mean reversion by looking at prior stock-selection skill versus top-level return.

So What
At Epsilon, we leverage these techniques to attempt to create predictive factors based upon the expressed beliefs (i.e., position-level holdings!) of all active managers, delivering that risk premia to our clients in liquid, transparent, and low-fee vehicles. We’ve run thousands of tests examining fascinating relationships like the simple analysis above. We want to understand relationships such as manager size and alpha-generating potential, who the “leaders” in the hedge fund world are, and if position-sizing alpha is a more persistent skill than security-selection alpha. So it’s easy for us to preach this type of assessment when it’s our livelihood. But the excuses behind complexity are increasingly invalid given the proliferation of tooling made available. If getting started is daunting, partnering with experts is the first step. It’s incumbent to at least try to engage, improving process and ultimately returns. After all, it’s all about the returns.
The information contained in this article was obtained from various sources that Epsilon believes to be reliable, but Epsilon does not guarantee its accuracy or completeness. The information and opinions contained on this site are subject to change without notice.
Neither the information nor any opinion contained on this site constitutes an offer, or a solicitation of an offer, to buy or sell any securities or other financial instruments, including any securities mentioned in any report available on this site.
The information contained on this site has been prepared and circulated for general information only and is not intended to and does not provide a recommendation with respect to any security. The information on this site does not take into account the financial position or particular needs or investment objectives of any individual or entity. Investors must make their own determinations of the appropriateness of an investment strategy and an investment in any particular securities based upon the legal, tax and accounting considerations applicable to such investors and their own investment objectives. Investors are cautioned that statements regarding future prospects may not be realized and that past performance is not necessarily indicative of future performance.